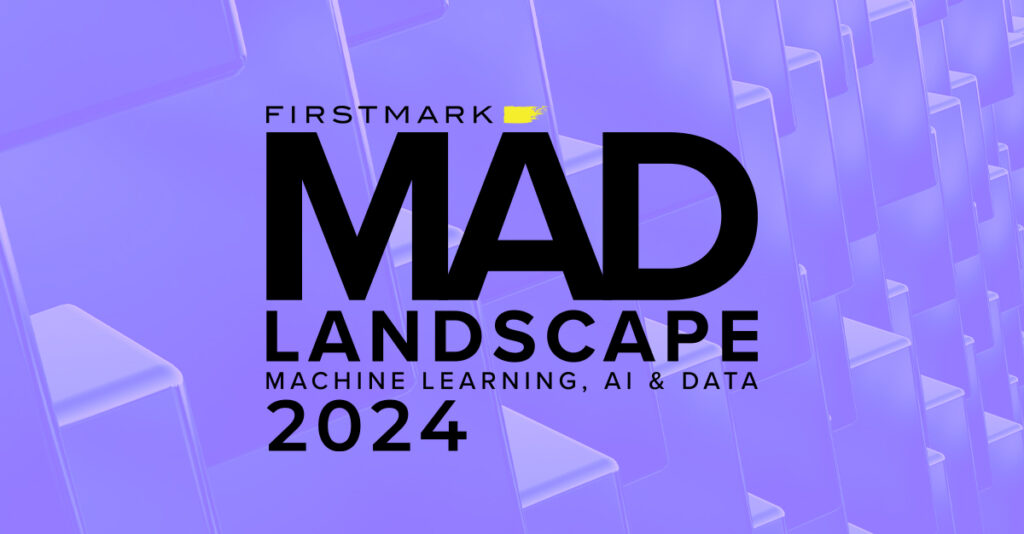
That is our tenth annual panorama and “state of the union” of the information, analytics, machine studying and AI ecosystem.
In 10+ years protecting the area, issues have by no means been as thrilling and promising as they’re right now. All tendencies and subtrends we described through the years are coalescing: knowledge has been digitized, in huge quantities; it may be saved, processed and analyzed quick and cheaply with fashionable instruments; and most significantly, it may be fed to ever-more performing ML/AI fashions which may make sense of it, acknowledge patterns, make predictions primarily based on it, and now generate textual content, code, photographs, sounds and movies.
The MAD (ML, AI & Information) ecosystem has gone from area of interest and technical, to mainstream. The paradigm shift appears to be accelerating with implications that go far past technical and even enterprise issues, and affect society, geopolitics and maybe the human situation. Maybe all of a sudden for some, it has develop into “all the things, all over the place unexpectedly”.
There are nonetheless many chapters to jot down within the multi-decade megatrend, nonetheless. As yearly, this submit is an try at making sense of the place we’re presently, throughout merchandise, corporations and business tendencies.
Listed here are the prior variations: 2012, 2014, 2016, 2017, 2018, 2019 (Half I and Half II), 2020, 2021 and 2023 (Half I, Half II, Half III, Half IV).
Our crew this 12 months was Aman Kabeer and Katie Mills (FirstMark), Jonathan Grana (Go Fractional) and Paolo Campos, main because of all. And an enormous thanks as nicely to CB Insights for offering the cardboard knowledge showing within the interactive model.
This annual state of the union submit is organized in three elements:
- Half: I: The panorama (PDF, Interactive model)
- Half II: 24 themes we’re serious about in 2024
- Half III: Financings, M&A and IPOs
PART I: THE LANDSCAPE
Hyperlinks
To see a PDF of the 2024 MAD Panorama in full decision (please zoom!), please CLICK HERE
To entry the interactive model of the 2024 MAD panorama, please CLICK HERE
Variety of corporations
The 2024 MAD panorama options 2,011 logos in whole.
That quantity is up from 1,416 final 12 months, with 578 new entrants to the map.
For reference, the very first model in 2012 has simply 139 logos.
The intensely (insanely?) crowded nature of the panorama primarily results from two back-to-back huge waves of firm creation and funding.
The primary wave was the 10-ish 12 months lengthy knowledge infrastructure cycle, which began with Large Information and ended with the Trendy Information Stack. The lengthy awaited consolidation in that area has not fairly occurred but, and the overwhelming majority of the businesses are nonetheless round.
The second wave is the ML/AI cycle, which began in earnest with Generative AI. As we’re within the early innings of this cycle, and most corporations are very younger, we have now been liberal in together with younger startups (an excellent variety of that are seed stage nonetheless) within the panorama.
Observe: these two waves are intimately associated. A core thought of the MAD Panorama yearly has been to indicate the symbiotic relationship between knowledge infrastructure (on the left facet); analytics/BI and ML/AI (within the center) and functions (on the suitable facet).
Whereas it will get tougher yearly to suit the ever-increasing variety of corporations on the panorama yearly, however in the end, one of the simplest ways to consider the MAD area is as an meeting line – a full lifecycle of knowledge from assortment to storage to processing to delivering worth by means of analytics or functions.
Two large waves + restricted consolidation = plenty of corporations on the panorama.
Fundamental adjustments in “Infrastructure” and “Analytics“
We’ve made only a few adjustments to the general construction of left facet of the panorama – as we’ll see beneath (Is the Trendy Information Stack lifeless?), this a part of the MAD panorama has seen lots much less warmth recently.
Some noteworthy adjustments: We renamed “Database Abstraction” to “Multi-Mannequin Databases & Abstractions”, to seize the rising wave round an all-in-one ‘Multi-Mannequin’ database group (SurrealDB*, EdgeDB); killed the “Crypto / Internet 3 Analytics” part we experimentally created final 12 months, which felt misplaced on this panorama; and eliminated the “Question Engine” part, which felt extra like part of a piece than a separate part (all the businesses in that part nonetheless seem on the panorama – Dremio, Starburst, PrestoDB and so on).
Fundamental adjustments in “Machine Studying & Synthetic Intelligence”
With the explosion of AI corporations in 2023, that is the place we discovered ourselves making by far essentially the most structural adjustments.
- Given the large exercise within the ‘AI enablement’ layer within the final 12 months, we added 3 new classes subsequent to MLOps:
- “AI Observability” is a brand new class this 12 months, with startups that assist take a look at, consider and monitor LLM functions
- “AI Developer Platforms” is shut in idea to MLOps however we needed to acknowledge the wave of platforms which can be wholly centered on AI utility improvement, specifically round LLM coaching, deployment and inference
- “AI Security & Safety” consists of corporations addressing considerations innate to LLMs, from hallucination to ethics, regulatory compliance, and so on
- If the very public beef between Sam Altman and Elon Musk has informed us something, it’s that the excellence between industrial and nonprofit is a essential one in relation to foundational mannequin builders. As such, we have now cut up what was beforehand “Horizontal AI/AGI” into two classes: “Business AI Analysis” and “Nonprofit AI Analysis”
- The ultimate change we made was one other nomenclature one, the place we amended “GPU Cloud” to mirror the addition of core infrastructure characteristic units made by lots of the GPU Cloud suppliers: “GPU Cloud / ML Infra”
Fundamental adjustments in “Functions”
- The most important replace right here is that…to utterly nobody’s shock…each application-layer firm is now a self-proclaimed “AI firm” – which, as a lot as we tried to filter, drove the explosion of recent logos you see on the suitable facet of the MAD panorama this 12 months
- Some minor adjustments on the construction facet:
- In “Horizontal Functions,” we added a “Presentation & Design” class
- We renamed “Search” to Search / Conversational AI” to mirror the rise of LLM-powered chat-based interface comparable to Perplexity.
- In “Trade”, we rebranded “Gov’t & Intelligence” to “Aerospace, Protection & Gov’t”
Fundamental adjustments in “Open Supply Infrastructure”
- We merged classes which have at all times been shut, making a single “Information Administration” class that spans each “Information Entry” and “Information Ops”
- We added an necessary new class, “Native AI” as builders sought to supply the infrastructure tooling to convey AI & LLMs to the native improvement age
PART II: 24 THEMES WE’RE THINKING ABOUT IN 2024
Issues in AI are each shifting so quick, and getting a lot protection, that it’s nearly unattainable to supply a totally complete “state of the union” of the MAD area, as we did in prior years.
So right here’s for a special format: in no specific order, listed below are 24 themes which can be high of thoughts and/or come up continuously in conversations. Some are pretty fleshed out ideas, some largely simply questions or thought experiments.
- Structured vs unstructured knowledge
That is partly a theme, partly one thing we discover ourselves mentioning lots in conversations to assist clarify the present tendencies.
So, maybe as an introduction to this 2024 dialogue, right here’s one necessary reminder upfront, which explains among the key business tendencies. Not all knowledge is identical. On the danger of grossly over-simplifying, there are two major households of knowledge, and round every household, a set of instruments and use instances has emerged.
- Structured knowledge pipelines: that’s knowledge that may match into rows and columns.
- For analytical functions, knowledge will get extracted from transactional databases and SaaS instruments, saved in cloud knowledge warehouses (like Snowflake), reworked, and analyzed and visualized utilizing Enterprise Intelligence (BI) instruments, largely for functions of understanding the current and the previous (what’s referred to as “descriptive analytics”). That meeting line is usually enabled by the Trendy Information Stack mentioned beneath, with analytics because the core use case.
- As well as, structured knowledge also can get fed in “conventional” ML/AI fashions for functions of predicting the longer term (predictive analytics) – for instance, which prospects are most probably to churn
- Unstructured knowledge pipelines: that’s the world of knowledge that usually doesn’t match into rows and columns comparable to textual content, photographs, audio and video. Unstructured knowledge is essentially what will get fed in Generative AI fashions (LLMs, and so on), each to coach and use (inference) them.
These two households of knowledge (and the associated instruments and corporations) are experiencing very totally different fortunes and ranges of consideration proper now.
Unstructured knowledge (ML/AI) is sizzling; structured knowledge (Trendy Information Stack, and so on) shouldn’t be.
- Is the Trendy Information Stack lifeless?
Not that way back (name it, 2019-2021), there wasn’t something sexier within the software program world than the Trendy Information Stack (MDS). Alongside “Large Information”, it was one of many uncommon infrastructure ideas to have crossed over from knowledge engineers to a broader viewers (execs, journalists, bankers).
The Trendy Information Stack principally coated the form of structured knowledge pipeline talked about above. It gravitated across the fast-growing cloud knowledge warehouses, with distributors positioned upstream from it (like Fivetran and Airbyte), on high of it (DBT) and downstream from it (Looker, Mode).
As Snowflake emerged as the most important software program IPO ever, curiosity within the MDS exploded, with rabid, ZIRP-fueled firm creation and VC funding. Complete classes grew to become overcrowded inside a 12 months or two – knowledge catalogs, knowledge observability, ETL, reverse ETL, to call just a few.
An actual resolution to an actual drawback, the Trendy Information Stack was additionally a advertising idea and a de-facto alliance amongst quite a few startups throughout the worth chain of knowledge.
Quick ahead to right now, the state of affairs could be very totally different. In 2023, we had previewed that the MDS was “beneath strain”, and that strain will solely proceed to accentuate in 2024.
The MDS is going through two key points:
- Placing collectively a Trendy Information Stack requires stitching collectively varied best-of-breed options from a number of unbiased distributors. Consequently, it’s pricey by way of cash, time and assets. This isn’t appeared upon favorable by the CFO workplace in a submit ZIRP price range lower period
- The MDS is now not the cool child on the block. Generative AI has stolen all the eye from execs, VCs and the press – and it requires the form of unstructured knowledge pipelines we talked about above.
Watch: MAD Podcast: Is the Trendy Stack Useless? With Tristan Helpful, CEO, dbt Labs (Apple, Spotify)
- Consolidation in knowledge infra, and the large getting greater
Given the above, what occurs subsequent in knowledge infra and analytics in 2024?
It might look one thing like this:
- Many startups in and across the Trendy Information Stack will aggressively reposition as “AI infra startups” and attempt to discover a spot within the Trendy AI Stack (see beneath). This can work in some instances, however going from structured to unstructured knowledge could require a elementary product evolution most often.
- The information infra business will lastly see some consolidation. M&A has been pretty restricted so far, however some acquisitions did occur in 2023, whether or not tuck-ins or medium-size acquisitions – together with Stemma (acquired by Teradata), Manta (acquired by IBM), Mode (acquired by Thoughtspot), and so on (see PART III beneath)
- There can be much more startup failure – as VC funding dried up, issues have gotten robust. Many startups have lower prices dramatically, however in some unspecified time in the future their money runway will finish. Don’t anticipate to see flashy headlines, however this may (sadly) occur.
- The larger corporations within the area, whether or not scale-ups or public corporations, will double down on their platform play and push exhausting to cowl ever extra performance. A few of will probably be by means of acquisitions (therefore the consolidation) however plenty of it can even be by means of homegrown improvement.
- Checking in on Databricks vs Snowflake
Talking of huge corporations within the area, let’s examine in on the “titanic shock” (see our MAD 2021 weblog submit) between the 2 key knowledge infra gamers, Snowflake and Databricks.
Snowflake (which traditionally comes from the structured knowledge pipeline world) stays an unimaginable firm, and one of many highest valued public tech shares (14.8x EV/NTM income as of the time of writing). Nonetheless, very like plenty of the software program business, its progress has dramatically slowed down – it completed fiscal 2024 with a 38% year-over-year product income progress, totaling $2.67 billion, projecting 22% NTM rev progress as of the time of writing). Maybe most significantly, Snowflake gives the look of an organization beneath strain on the product entrance – it’s been slower to embrace AI, and relatively much less acquisitive. The current, and considerably abrupt, CEO transition is one other fascinating knowledge level.
Databricks (which traditionally comes from the unstructured knowledge pipeline and machine studying world) is experiencing all-around sturdy momentum, reportedly (because it’s nonetheless a personal firm) closing FY’24 with $1.6B in income with 50%+ progress. Importantly, Databricks is rising as a key Generative AI participant, each by means of acquisitions (most notably, MosaicML for $1.3B) and homegrown product improvement – at the start as a key respiratory for the form of unstructured knowledge that feeds LLMs, but additionally as creator of fashions, from Dolly to DBRX, a brand new generative AI mannequin the corporate simply introduced on the time of writing.
The foremost new evolution within the Snowflake vs Databricks rivalry is the launch of Microsoft Cloth. Introduced in Might 2023, it’s an end-to-end, cloud-based SaaS platform for knowledge and analytics. It integrates plenty of Microsoft merchandise, together with OneLake (open lakehouse), PowerBI and Synapse Information Science, and covers principally all knowledge and analytics workflows, from knowledge integration and engineering to knowledge science. As at all times for giant firm product launches, there’s a niche between the announcement and the fact of the product, however mixed with Microsoft’s main push in Generative AI, this might develop into a formidable menace (as a further twist to the story, Databricks largely sits on high of Azure).
- BI in 2024, and Is Generative AI about to remodel knowledge analytics?
Of all elements of the Trendy Information Stack and structured knowledge pipelines world, the class that has felt essentially the most ripe for reinvention is Enterprise Intelligence. We highlighted within the 2019 MAD how the BI business had nearly completely consolidated, and talked in regards to the emergence of metrics shops within the 2021 MAD.
The transformation of BI/analytics has been slower than we’d have anticipated. The business stays largely dominated by older merchandise, Microsoft’s PowerBI, Salesforce’s Tableau and Google’s Looker, which typically get bundled in at no cost in broader gross sales contracts. Some extra consolidation occurred (Thoughtspot acquired Mode; Sisu was quietly acquired by Snowflake). Some younger corporations are taking progressive approaches, whether or not scale-ups (see dbt and their semantic layer/MetricFlow) or startups (see Hint* and their metrics tree), however they’re typically early within the journey.
Along with probably enjoying a robust position in knowledge extraction and transformation, Generative AI might have a profound affect by way of superpowering and democratizing knowledge analytics.
There’s actually been plenty of exercise. OpenAI launched Code Interpreter, later renamed to Superior Information Evaluation. Microsoft launched a Copilot AI chatbot for finance employees in Excel. Throughout cloud distributors, Databricks, Snowflake, open supply and a considerable group of startups, lots of people are engaged on or have launched “textual content to SQL” merchandise, to assist run queries into databases utilizing pure language.
The promise is each thrilling and probably disruptive. The holy grail of knowledge analytics has been its democratization. Pure language, if it had been to develop into the interface to notebooks, databases and BI instruments, would allow a wider group of individuals to do evaluation.
Many individuals within the BI business are skeptical, nonetheless. The precision of SQL and the nuances of understanding the enterprise context behind a question are thought of large obstacles to automation.
- The Rise of the Trendy AI Stack
A variety of what we’ve mentioned up to now needed to do with the world of structured knowledge pipelines.
As talked about, the world of unstructured knowledge infrastructure is experiencing a really totally different second. Unstructured knowledge is what feeds LLMs, and there’s rabid demand for it. Each firm that’s experimenting or deploying Generative AI is rediscovering the outdated cliche: “knowledge is the brand new oil”. Everybody needs the facility of LLMs, however skilled on their (enterprise) knowledge.
Corporations large and small have been dashing into the chance to supply the infrastructure of Generative AI.
A number of AI scale-ups have been aggressively evolving their choices to capitalize on market momentum – everybody from Databricks (see above) to Scale AI (which developed their labeling infrastructure, initially developed for the self-driving automotive market, to accomplice as an enterprise knowledge pipeline with OpenAI and others) to Dataiku* (which launched their LLM Mesh to allow International 2000 corporations to seamlessly work throughout a number of LLM distributors and fashions).
In the meantime a brand new era of AI infra startups is rising, throughout quite a few domains, together with:
- Vector databases, which retailer knowledge in a format (vector embeddings) that Generative AI fashions can devour. Specialised distributors (Pinecone, Weaviate, Chroma, Qudrant and so on) have had a banner 12 months, however some incumbent database gamers (MongoDB) had been additionally fast to react and add vector search capabilities.
- Frameworks (LlamaIndex, Langchain and so on), which join and orchestrate all of the shifting items
- Guardrails, which sit between an LLM and customers and ensure the mannequin gives outputs that observe the group’s guidelines.
- Evaluators which assist take a look at, analyze and monitor Generative AI mannequin efficiency, a tough drawback as demonstrated by the overall mistrust in public benchmarks
- Routers, which assist direct person queries throughout totally different fashions in actual time, to optimize efficiency, price and person expertise
- Value guards, whichhelp monitor the prices of utilizing LLMs
- Endpoints, successfully APIs that summary away the complexities of underlying infrastructure (like fashions)
We’ve been resisting utilizing the time period “Trendy AI Stack”, given the historical past of the Trendy Information Stack.
However the expression captures the various parallels: lots of these startups are the “sizzling corporations” of the day, similar to MDS corporations earlier than them, they have a tendency to journey in pack, forging advertising alliances and product partnerships. And maybe there
And this new era of AI infra startups goes to face among the identical challenges as MDS corporations earlier than them: are any of these classes sufficiently big to construct a multi-billion greenback firm? Which half will large corporations (largely cloud suppliers, but additionally Databricks and Snowflake) find yourself constructing themselves?
WATCH – we featured many rising startups on the MAD Podcast:
- The place are we within the AI hype cycle?
AI has a multi decade-long historical past of AI summers and winters. Simply within the final 10-12 years, that is the third AI hype cycle we’ve skilled: there was one in 2013-2015 after deep studying got here to the limelight submit ImageNet 2012; one other one someday round 2017-2018 throughout the chatbot growth and the rise of TensorFlow; and now since November 2022 with Generative AI.
This hype cycle has been notably intense, to the purpose of feeling like an AI bubble, for quite a few causes: the expertise is extremely spectacular; it is extremely visceral and crossed over to a broad viewers past tech circles; and for VCs sitting on plenty of dry powder, it’s been the solely recreation on the town as nearly all the things else in expertise has been depressed.
Hype has introduced all the same old advantages (“nothing nice has ever been achieved with out irrational exuberance”, “let a 1000 flowers bloom” part, with plenty of cash accessible for formidable tasks) and noise (everyone seems to be an AI knowledgeable in a single day, each startup is an AI startup, too many AI conferences/podcasts/newsletters… and dare we are saying, too many AI market maps???).
The principle concern of any hype cycle is the inevitable blowback.
There’s a good quantity of “quirkiness” and danger constructed into this market part: the poster-child firm for the area has a really uncommon authorized and governance construction; there are plenty of “compute for fairness” offers taking place (with potential round-tripping) that aren’t totally understood or disclosed; plenty of high startups are run by groups of AI researchers; and plenty of VC dealmaking is harking back to the ZIRP occasions: “land grabs”, large rounds and eye-watering valuations for very younger corporations.
There actually have been cracks in AI hype (see beneath), however we’re nonetheless in a part the place each week a brand new factor blows everybody’s minds. And information just like the reported $40B Saudi Arabia AI fund appear to level that cash flows into the area are usually not going to cease anytime quickly.
- Experiments vs actuality: was 2023 a headfake?
Associated to the above – given the hype, how a lot has been actual up to now, vs merely experimental?
2023 was an motion packed 12 months: a) each tech vendor rushed to incorporate Generative AI of their product providing, b) each International 2000 board mandated their groups to “do AI”, and a few enterprise deployments occurred a file velocity, together with at corporations in regulated industries like Morgan Stanley and Citibank and c) after all, customers confirmed rabid curiosity for Generative AI apps.
Consequently, 2023 was a 12 months of huge wins: OpenAI reached $2B in annual run charge; Anthropic grew at a tempo that allowed it to forecast $850M in revenues for 2024; Midjourney grew to $200M in income with no funding and a crew of 40; Perplexity AI went from 0 to 10 million month-to-month energetic customers, and so on.
Ought to we be cynical? Some considerations:
- Within the enterprise, plenty of the spend was on proof of ideas, or simple wins, usually popping out of innovation budgets.
- How a lot was pushed by executives eager to not seem flat-footed, vs fixing precise enterprise issues?
- In shopper, AI apps present excessive churn. How a lot was it mere curiosity?
- Each of their private {and professional} lives, many report not being completely certain what to do with Generative AI apps and merchandise
- Ought to we view Inflection AI’s determination to fold shortly an admission that the world doesn’t want one more AI chatbot, and even LLM supplier?
- LLM corporations: perhaps not so commoditized in any case?
Billions of enterprise capital and company cash are being invested in foundational mannequin corporations.
Therefore everybody’s favourite query within the final 18 months: are we witnessing a phenomenal incineration of capital into in the end commoditized merchandise? Or are these LLM suppliers the brand new AWS, Azure and GCP?
A troubling truth (for the businesses concerned) is that no LLM appears to be constructing a sturdy efficiency benefit. On the time of writing, Claude 3 Sonnet and Gemini Professional 1.5 carry out higher than GPT-4 which performs higher than Gemini 1.0 Extremely, and so forth and so forth – however this appears to alter each few weeks. Efficiency can also fluctuate – ChatGPT in some unspecified time in the future “misplaced its thoughts” and “obtained lazy”, quickly.
As well as, open supply fashions (Llama 3, Mistral and others like DBRX) are shortly catching up by way of efficiency.
Individually – there are much more LLM suppliers available on the market than might have appeared at first. A few years in the past, the prevailing narrative was that there could possibly be just one or two LLM corporations, with a winner-take-all dynamic – partially as a result of there was a tiny variety of individuals world wide with the mandatory experience to scale Transformers.
It turns on the market are extra succesful groups than first anticipated. Past OpenAI and Anthropic, there are a variety of startups doing foundational AI work – Mistral, Cohere, Adept, AI21, Imbue, 01.AI to call just a few – after which after all the groups at Google, Meta, and so on.
Having mentioned that – up to now the LLM suppliers appear to be doing simply advantageous. OpenAI and Anthropic revenues are rising at extraordinary charges, thanks very a lot. Perhaps the LLM fashions do get commoditized, the LLM corporations nonetheless have an immense enterprise alternative in entrance of them. They’ve already develop into “full stack” corporations, providing functions and tooling to a number of audiences (shopper, enterprise, builders), on high of the underlying fashions.
Maybe the analogy with cloud distributors is certainly fairly apt. AWS, Azure and GCP appeal to and retain prospects by means of an utility/tooling layer and monetize by means of a compute/storage layer that’s largely undifferentiated.
WATCH:
- LLMs, SLMs and a hybrid future
For all the joy about Giant Language Fashions, one clear pattern of the previous couple of months has been the acceleration of small language fashions (SLMs), comparable to Llama-2-13b from Meta, Mistral-7b and Mixtral 8x7b from Mistral and Phi-2 and Orca-2 from Microsoft.
Whereas the LLMs are getting ever greater (GPT-3 reportedly having 175 billion parameters, GPT-4 reportedly having 1.7 trillion, and the world ready for an much more huge GPT-5), SLMs have gotten a robust various for a lot of use instances are they’re cheaper to function, simpler to finetune, and infrequently supply sturdy efficiency.
One other pattern accelerating is the rise of specialised fashions, centered on particular duties like coding (Code-Llama, Poolside AI) or industries (e.g. Bloomberg’s finance mannequin, or startups Orbital Supplies constructing modelsl for materials sciences, and so on).
As we’re already seeing throughout quite a few enterprise deployments, the world is shortly evolving in direction of hybrid architectures, combining a number of fashions.
Though costs have been taking place (see beneath), large proprietary LLMs are nonetheless very costly, expertise latency issues, and rso customers/prospects will more and more be deploying mixtures of fashions, large and small, industrial and open supply, basic and specialised, to satisfy their particular wants and value constraints.
Watch: MAD Podcast with Eiso Kant, CTO, Poolside AI (additionally: Apple Podcasts, Spotify)
- Is conventional AI lifeless?
A humorous factor occurred with the launch of ChatGPT: a lot of the AI that had been deployed up till then obtained labeled in a single day as “Conventional AI”, in distinction to “Generative AI”.
This was just a little little bit of a shock to many AI practitioners and corporations that up till then had been thought of to be doing modern work, because the time period “conventional” clearly suggests an impending wholesale alternative of all types of AI by the brand new factor.
The fact is much more nuanced. Conventional AI and Generative AI are in the end very complementary as they deal with various kinds of knowledge and use instances.
What’s now labeled as “conventional AI”, or sometimes as “predictive AI” or “tabular AI”, can be very a lot a part of fashionable AI (deep studying primarily based). Nonetheless, it typically focuses on structured knowledge (see above), and issues comparable to suggestions, churn prediction, pricing optimization, stock administration. “Conventional AI” has skilled large adoption within the final decade, and it’s already deployed at scale in manufacturing in hundreds of corporations world wide.
In distinction, Generative AI largely operates on unstructured knowledge (textual content, picture, movies, and so on.). Is exceptionally good at a special class of issues (code era, picture era, search, and so on).
Right here as nicely, the longer term is hybrid: corporations will use LLMs for sure duties, predictive fashions for different duties. Most significantly, they are going to usually mix them – LLMs is probably not nice at offering a exact prediction, like a churn forecast, however you can use an LLM that calls on the output of one other mannequin which is targeted on offering that prediction, and vice versa.
- Skinny wrappers, thick wrappers and the race to be full stack
“Skinny wrappers” was the dismissive time period everybody cherished to make use of in 2023. It’s exhausting to construct lengthy lasting worth and differentiation in case your core capabilities are offered by another person’s expertise (like OpenAI), the argument goes. And reviews just a few months in the past that startups like Jasper had been operating into difficulties, after experiencing a meteoric income rise, appear to corroborate that line of pondering.
The fascinating query is what occurs over time, as younger startups construct extra performance. Do skinny wrappers develop into thick wrappers?
In 2024, it looks like thick wrappers have a path in direction of differentiation by:
- Specializing in a particular drawback, usually vertical – as something too horizontal runs the danger of being within the “kill zone” of Large Tech
- Constructing workflow, collaboration and deep integrations, which can be particular to that drawback
- Doing plenty of work on the AI mannequin degree – whether or not finetuning fashions with particular datasets or creating hybrid techniques (LLMs, SLMs, and so on) tailor-made for his or her particular enterprise
In different phrases, they are going to should be each slender and “full stack” (each functions and infra).
- Fascinating areas to observe in 2024: AI brokers, Edge AI
There’s been loads of pleasure over the past 12 months across the idea of AI brokers – principally the final mile of an clever system that may execute duties, usually in a collaborative method. This could possibly be something from serving to to e book a visit (shopper use case) to robotically operating full SDR campaigns (productiveness use case) to RPA-style automation (enterprise use case).
AI brokers are the holy grail of automation – a “textual content to motion” paradigm the place AI simply will get stuff performed for us.
Each few months, the AI world goes loopy for an agent-like product, from BabyAGI final 12 months to Devin AI (an “AI software program engineer”) only recently. Nonetheless, on the whole, a lot of this pleasure has confirmed untimely so far. There’s plenty of work to be performed first to make Generative much less brittle and extra predictable, earlier than advanced techniques involving a number of fashions can work collectively and take precise actions on our behalf. There are additionally lacking elements – comparable to the necessity to construct extra reminiscence into AI techniques. Nonetheless, anticipate AI brokers to be a very thrilling space within the subsequent 12 months or two.
One other fascinating space is Edge AI. As a lot as there’s a large marketplace for LLMs that run at huge scale and delivered as finish factors, a holy grail in AI has been fashions that may run domestically on a tool, with out GPUs, specifically telephones, but additionally clever, IoT-type gadgets. The area could be very vibrant: Mixtral, Ollama, Llama.cpp, Llamafile, GPT4ALL (Nomic). Google and Apple are additionally more likely to be more and more energetic.
- Is Generative AI heading in direction of AGI, or in direction of a plateau?
It’s nearly a sacrilegious query to ask given all of the breathless takes on AI, and the unimaginable new merchandise that appear to return out each week – however is there a world the place progress in Generative AI slows down relatively than accelerates all the way in which to AGI? And what would that imply?
The argument is twofold: a) foundational fashions are a brute power train, and we’re going to expire of assets (compute, knowledge) to feed them, and b) even when we don’t run out, in the end the path to AGI is reasoning, which LLMs are usually not able to doing.
Apparently, this is kind of the identical dialogue because the business was having 6 years in the past, as we described in a 2018 weblog submit. Certainly what appears to have modified largely is the sheer quantity of knowledge and compute we’ve thrown at (more and more succesful) fashions.
How shut we’re from any form of “operating out” could be very exhausting to evaluate. The frontier for “operating out of compute” appears to be pushed again additional day-after-day. NVIDIA’s just lately introduced Blackwell GPU system, and the corporate says it might probably deploy a 27 trillion parameter mannequin (vs 1.7 trillion for GPT-4).
The information half is advanced – there’s a extra tactical query round operating out of legally licensed knowledge (see all of the OpenAI licensing offers), and a broader query round operating out of textual knowledge, on the whole. There may be actually plenty of work taking place round artificial knowledge. Yann LeCun mentioned how taking fashions to the following degree would in all probability require them to have the ability to ingest a lot richer video enter, which isn’t but doable.
From the slender perspective of individuals within the startup ecosystem (founders, traders), maybe the query issues much less, within the medium time period – if Generative AI stopped making progress tomorrow, we’d nonetheless have years of alternative forward deploying what we presently have throughout verticals and use instances.
- The GPU wars (is NVIDIA overvalued?)
Are we within the early innings of an enormous cycle the place compute turns into essentially the most valuable commodity on the planet, or dramatically over-building GPU manufacturing in a manner that’s certain to result in an enormous crash?
As just about the one recreation on the town in relation to Generative AI-ready GPUs, NVIDIA actually has been having fairly the second, with a share worth up five-fold to a $2.2 trillion valuation, and whole gross sales three-fold since late 2022, huge pleasure round its earnings and Jensen Huang at GTC rivaling Taylor Swift for the most important occasion of 2024.
Maybe this was additionally partially as a result of it was the last word beneficiary of all of the billions invested by VCs in AI?
Regardless, for all its plain prowess as an organization, NVIDIA’s fortunes can be tied to how sustainable the present gold rush will turn into. {Hardware} is tough, and predicting with accuracy what number of GPUs should be manufactured by TSMC in Taiwan is a troublesome artwork.
As well as, competitors is making an attempt its greatest to react, from AMD to Intel to Samsung; startups (like Groq or Cerebras) are accelerating, and new ones could also be fashioned, like Sam Altman’s rumored $7 trillion chip firm. A brand new coalition of tech corporations together with Google, Intel and Qualcomm is making an attempt to go after NVIDIA’s secret weapon: its CUDA software program that retains builders tied to Nvidia chips.
Our take: Because the GPU scarcity subsides, there could also be short-to medium time period downward strain on NVIDIA, however the long run for AI chips producers stays extremely shiny.
- Open supply AI: an excessive amount of of an excellent factor?
This one is simply to stir a pot just a little bit. We’re large followers of open supply AI, and clearly this has been an enormous pattern of the final 12 months or so. Meta made a serious push with its Llama fashions, France’s Mistral went from controversy fodder to new shining star of Generative AI, Google launched Gemma, and HuggingFace continued its ascension because the ever so vibrant residence of open supply AI, internet hosting a plethora of fashions. Among the most progressive work in Generative AI has been performed within the open supply neighborhood.
Nonetheless, there’s additionally a basic feeling of inflation permeating the neighborhood. A whole bunch of hundreds of open supply AI fashions are actually accessible. Many are toys or weekend tasks. Fashions go up and down the rankings, a few of them experiencing meteoric rises by Github star requirements (a flawed metric, however nonetheless) in just some days, solely to by no means rework into something notably usable. It’s been dizzying for a lot of.
Our take: the market can be self-correcting, with an influence regulation of profitable open-source tasks.
- How a lot does AI truly price?
The economics of Generative AI is a fast-evolving subject. And never surprisingly, plenty of the way forward for the area revolves round it – for instance, can one critically problem Google in search, if the price of offering AI-driven solutions is considerably increased than the price of offering ten blue hyperlinks? And may software program corporations actually be AI-powered if the inference prices eat up chunks of their gross margin?
The excellent news, in the event you’re a buyer/person of AI fashions: we appear to be within the early part of a race to the underside on the worth facet, which is occurring quicker than one could have predicted. One key driver has been the parallel rise of open supply AI (Mistral and so on) and industrial inference distributors (Collectively AI, Anyscale, Replit) taking these open fashions and serving them as finish factors. There are little or no switching prices for purchasers (apart from the complexity of working with totally different fashions producing totally different outcomes), and that is placing strain on OpenAI and Anthropic. An instance of this has been the numerous price drops for embedding fashions the place a number of distributors (OpenAI, Collectively AI and so on) dropped costs on the identical time.
From a vendor perspective, the prices of constructing and serving AI stay very excessive. It was reported within the press that Anthropic spent greater than half of the income it generated paying cloud suppliers like AWS and GCP to run its LLMs. There’s the price of licensing offers with publishers as nicely
On the plus facet, perhaps all of us as customers of Generative applied sciences ought to simply benefit from the explosion of VC-subsidized free companies:
Watch: MAD Podcast with Nomic
- Large corporations and the shifting political financial system of AI: Has Microsoft gained?
This was one of many first questions everybody requested in late 2022, and it’s much more high of thoughts in 2024: will Large Tech seize many of the worth in Generative AI?
AI rewards measurement – extra knowledge, extra compute, extra AI researchers tends to yield extra energy. Large Tech has been keenly conscious of this, and in contrast to incumbents in prior platform shifts, intensely reactive to the potential disruption forward.
Amongst Large Tech corporations, it actually looks like Microsoft has been enjoying 4-D chess. There’s clearly the connection with OpenAI, however Microsoft additionally partnered with open supply rival Mistral. It invested in ChatGPT rival Inflection AI (Pi), solely to acqui-hire it in spectacular vogue just lately. And in the end, all these partnerships appear to solely create extra want for Microsoft’s cloud compute – Azure income grew 24% year-over-year to achieve $33 billion in Q2 2024, with 6 factors of Azure cloud progress attributed to AI companies.
In the meantime, Google and Amazon have partnered with and invested in OpenAI rival Anthropic (on the time of writing, Amazon simply dedicated one other $2.75B to the corporate, within the 2nd tranche of its deliberate $4B funding). Amazon additionally partnered with open supply platform Hugging Face. Google and Apple are reportedly discussing an integration of Gemini AI in Apple merchandise. Meta is presumably under-cutting everybody by going full hog on open supply AI. Then there may be all the things taking place in China.
The apparent query is how a lot room there may be for startups to develop and succeed. A primary tier of startups (OpenAI and Anthropic, primarily, with maybe Mistral becoming a member of them quickly) appear to have struck the suitable partnerships, and reached escape velocity. For lots of different startups, together with very nicely funded ones, the jury continues to be very a lot out.
Ought to we learn in Inflection AI’s determination to let itself get acquired, and Stability AI’s CEO troubles an admission that industrial traction has been tougher to attain for a bunch of “second tier” Generative AI startups?
- Fanboying OpenAI – or not?
OpenAI continues to fascinate – the $86B valuation, the income progress, the palace intrigue, and Sam Altman being the Steve Jobs of this era:
A few fascinating questions:
Is OpenAI making an attempt to do an excessive amount of? Earlier than all of the November drama, there was the OpenAI Dev Day, throughout which OpenAI made it clear that it was going to do *all the things* in AI, each vertically (full stack) and horizontally (throughout use instances): fashions + infrastructure + shopper search + enterprise + analytics + dev instruments + market, and so on. It’s not an unprecedented technique when a startup is an early chief in an enormous paradigm shift with de facto limitless entry to capital (Coinbase kind of did it in crypto). However will probably be fascinating to observe: whereas it will actually simplify the MAD Panorama, it’s going to be a formidable execution problem, notably in a context the place competitors has intensified. From ChatGPT laziness points to the underwhelming efficiency of its market effort counsel that OpenAI shouldn’t be proof against the enterprise regulation of gravity.
Will OpenAI and Microsoft break up? The connection with Microsoft has been fascinating – clearly Microsoft’s help has been an enormous increase for OpenAI by way of assets (together with compute) and distribution (Azure within the enterprise), and the transfer was broadly considered as a grasp transfer by Microsoft within the early days of the Generative AI wave. On the identical time, Microsoft has made it clear that it’s not depending on OpenAI (has all of the code, weights, knowledge), it has partnered with opponents (e.g. Mistral), and thru the Inflection AI acqui-hire it now has significantly beefed up its AI analysis crew.
In the meantime, will OpenAI wish to proceed being single threaded in a partnership with Microsoft, vs being deployed on different clouds?
Given OpenAI’s huge ambitions, and Microsoft goal at international domination, at what level do each corporations conclude that they’re extra opponents than companions?
- Will 2024 be the 12 months of AI within the enterprise?
As talked about above, 2023 within the enterprise felt like a type of pivotal years the place everybody scrambles to embrace a brand new pattern, however nothing a lot truly occurs, past some proof-of-concepts.
Maybe the most important winners of Generative AI in 2023 had been the Accentures of the world, which reportedly generated $2B in charges for AI consulting.
Regardless, there’s large hope that 2024 goes to be an enormous 12 months for AI within the enterprise – or a minimum of for Generative AI, as conventional AI already has a major footprint there already (see above).
However we’re early in answering among the key questions International 2000-type corporations face:
What are the use instances? The low hanging fruit use instances up to now have been largely a) code era co-pilots for developer groups, b) enterprise information administration (search, textual content summarization, translation, and so on), and c) AI chatbots for customer support (a use case that pre-dates Generative AI). There are actually others (advertising, automated SDRs and so on) however there’s lots to determine (co-pilot mode vs full automation and so on).
What instruments ought to we decide? As per the above, it looks like the longer term is hybrid, a mixture of economic distributors and open supply, large and small fashions, horizontal and vertical GenAI instruments. However the place does one begin?
Who can be deploying and sustaining the instruments? There’s a clear talent scarcity in International 2000 corporations. For those who thought recruiting software program builders was exhausting, simply attempt to recruit machine studying engineers.
How will we make sure that they don’t hallucinate? Sure there’s an incredible quantity of labor being performed round RAG and guardrails and evaluations and so on, however the chance {that a} Generative AI instrument could also be plain fallacious, and the broader query that we don’t actually understand how Generative AI fashions work, are large issues within the enterprise.
What’s the ROI? Giant tech corporations have been early in leveraging Generative AI for their very own wants, and so they’re exhibiting fascinating early knowledge. Of their earnings name, Palo Alto Networks talked about roughly halving the price of their T&E servicing, and ServiceNow talked about rising our developer innovation velocity by 52%, however we’re early in understanding the price / return equation for Generative AI within the enterprise.
The excellent news for Generative AI distributors is that there’s loads of curiosity from enterprise prospects to allocate price range (importantly, now not “innovation” budgets however precise OpEx price range, presumably re-allocated from different locations) and assets to figuring it out. However we’re in all probability speaking a few 3-5 12 months deployment cycle, relatively than one.
WATCH: MAD Podcast with Florian Douetteau, CEO, Dataiku
- Is AI going to kill SaaS?
This was one of many fashionable concepts of the final 12 months.
One model of the query: AI makes it 10x to code, so with just some common builders, you’ll be capable of create a custom-made model of a SaaS product, tailor-made to your wants. Why pay some huge cash to a SaaS supplier when you’ll be able to construct your individual.
One other model of the query: the longer term is one AI intelligence (presumably fabricated from a number of fashions) that runs your complete firm with a sequence of brokers. You now not purchase HR software program, finance software program or gross sales software program as a result of the AI intelligence does all the things, in a totally automated and seamless manner.
We appear to be considerably distant from each of these tendencies truly taking place in any form of full-fledged method, however as everyone knows, issues change very quick in AI.
Within the meantime, it looks like a possible model of the longer term is that SaaS merchandise are going to develop into extra highly effective as AI will get constructed into each one among them.
- Is AI going to kill enterprise capital?
Leaving apart the (ever-amusing) subject of whether or not AI might automate enterprise capital, each by way of firm choice, and post-investment value-add, there’s an fascinating sequence of questions round whether or not the asset class is correctly-sized for the AI platform shift:
Is Enterprise Capital too small? The OpenAIs of the world have wanted to lift billions of {dollars}, and may have to lift many extra billions. A variety of these billions have been offered by large companies like Microsoft – in all probability largely within the type of compute-for-equity offers. In fact, many VCs have invested in large foundational mannequin corporations, however at a minimal, these investments are a transparent departure from the standard VC software program investing mannequin. Maybe AI investing goes to require mega-sized VC funds – on the time of writing, Saudi Arabia appears to be about to launch a $40B AI fund in collaboration with US VC companies.
Is Enterprise Capital too large? For those who imagine that AI goes to 10x our productiveness, together with tremendous coders and automatic SDR brokers and automatic advertising creation, then we’re about to witness the start of a complete era of fully-automated corporations run by skeleton groups (or perhaps only one solo-preneur) that would theoretically attain a whole lot of thousands and thousands in revenues (and go public)?
Does a $100M ARR firm run by a solo-preneur want enterprise capital at any level in its journey?
- Will AI revive shopper?
Shopper has been in search of its subsequent wind because the social media and cellular days. Generative AI could very nicely be it.
Some fascinating areas (amongst many others):
Search: for the primary time in a long time, Google’s search monopoly has some early, however credible opponents. A handful of startups like Perplexity AI and You.com are main the evolution from search engine to reply engine.
AI companions: past the dystopian points, what if each human had an infinitely affected person and useful companion attuned to 1’s particular wants, whether or not for information, leisure or remedy
AI {hardware}: Humane, Rabbit, VisionPro are thrilling entries in shopper {hardware}
Hyper-personalized leisure: what new types of leisure and artwork will we invent as Generative AI powered instruments maintain getting higher (and cheaper)?
Watch:
- AI and blockchain: BS, or thrilling?
I do know, I do know. The intersection of AI and crypto looks like good fodder for X/Twitter jokes.
Nonetheless, it’s an plain concern that AI is getting centralized in a handful of corporations which have essentially the most compute, knowledge and AI expertise – from Large Tech to the famously-not-open OpenAI. In the meantime, the very core of the blockchain proposition is to allow the creation of decentralized networks that enable individuals to share assets and belongings. There may be fertile floor for exploration there, a subject we began exploring years in the past (presentation).
Quite a few AI-related crypto tasks have skilled noticeable acceleration, together with Bittensor* (decentralized machine intelligence platform), Render (decentralized GPU rendering platform), Arweave (decentralized knowledge platform).
Whereas we didn’t embody a crypto part on this 12 months’s MAD Panorama, that is an fascinating space to observe.
Now, as at all times, the query is whether or not the crypto business will be capable of assist itself, and never devolve into a whole lot of AI-related memecoins, pump-and-dump schemes and scams.
PART III: FINANCINGS, M&A AND IPOS
Financings
The present financing setting is among the “story of two markets” conditions, the place there’s A, and all the things else.
The general funding continued to falter, declining 42% to $248.4B in 2023. The primary few months of 2024 are exhibiting some doable inexperienced shoots, however as of now the pattern has been kind of the identical.
Information infrastructure, for all the explanations described above, noticed little or no funding exercise, with Sigma Computing and Databricks being among the uncommon exceptions.
Clearly, AI was a complete totally different story.
The inescapable traits of the AI funding market have been:
- A big focus of capital in a handful of startups, specifically OpenAI, Anthropic, Inflection AI, Mistral, and so on.
- A disproportionate degree of exercise from company traders. The three most energetic AI traders in 2023 had been Microsoft, Google and NVIDIA
- Some murkiness within the above company offers about what quantity is precise money, vs “compute for fairness”
Some noteworthy offers since our 2023 MAD, in tough chronological order (not an exhaustive checklist!):
OpenAI, a (or the?) foundational mannequin developer, raised $10.3B throughout two rounds, now valued at $86B; Adept, one other foundational mannequin developer, raised $350M at a $1B valuation; AlphaSense, a market analysis platform for monetary companies, raised $475M throughout two rounds, now valued at $2.5B, Anthropic, one more foundational mannequin developer, raised $6.45B over three rounds, at a $18.4B valuation; Pinecone, a vector database platform, raised $100M at a $750M valuation; Celestial AI, an optical interconnect expertise platform for reminiscence and compute, raised $275M throughout two rounds; CoreWeave, a GPU Cloud supplier, raised $421M at a $2.5B valuation; Lightmatter, developer of a light-powered chip for computing, raised $308M throughout two rounds, now valued at $1.2B; Sigma Computing, a cloud-hosted knowledge analytics platform, raised $340M at a $1.1B valuation; Inflection, one other foundational mannequin developer, raised $1.3B at a $4B valuation; Mistral, a foundational mannequin developer, raised $528M throughout two rounds, now valued at $2B; Cohere, (shock) a foundational mannequin developer, raised $270M at a $2B valuation; Runway, a generative video mannequin developer, raised $191M at a $1.5B valuation; Synthesia*, a video era platform for enterprise, raised $90M at a $1B valuation; Hugging Face, a machine studying and knowledge science platform for working with open supply fashions, raised $235M at a $4.5B valuation; Poolside, a foundational mannequin developer particularly for code era and software program improvement, raised $126M; Modular, an AI improvement platform, raised $100M at a $600M valuation; Imbue, an AI agent developer, raised $212M; Databricks, supplier of knowledge, analytics and AI options, raised $684M at a $43.2B valuation; Aleph Alpha, one other foundational mannequin developer, raised $486M; AI21 Labs, a foundational mannequin developer, raised $208M at a $1.4B valuation; Collectively, a cloud platform for generative AI improvement, raised $208.5M throughout two rounds, now valued at $1.25B; VAST Information, a knowledge platform for deep studying, raised $118M at a $9.1B valuation; Defend AI, an AI pilot developer for the aerospace and protection business, raised $500M at a $2.8B valuation; 01.ai, a foundational mannequin developer, raised $200M at a $1B valuation; Hadrian, a producer of precision element factories for aerospace and protection, raised $117M; Sierra AI, an AI chatbot developer for customer support / expertise, raised $110M throughout two rounds; Glean, an AI-powered enterprise search platform, raised $200M at a $2.2B valuation; Lambda Labs, a GPU Cloud supplier, raised $320M at a $1.5B valuation; Magic, a foundational mannequin developer for code era and software program improvement, raised $117M at a $500M valuation.
M&A, Take Privates
The M&A market has been pretty quiet because the 2023 MAD.
A variety of conventional software program acquirers had been centered on their very own inventory worth and general enterprise, relatively than actively in search of acquisition alternatives.
And the notably strict antitrust setting has made issues trickier for potential acquirers – resulting in much less offers and a few contortionist workouts just like the Inflection AI acquisition by Microsoft.
Personal fairness companies have been moderately energetic, looking for lower cost alternatives within the harder market.
Some noteworthy transactions involving corporations which have appeared through the years on the MAD panorama (so as of scale):
Broadcom, a semiconductor producer, acquired VMWare, a cloud computing firm, for $69B; Cisco, a networking and safety infrastructure firm, acquired Splunk, a monitoring and observability platform, for $28B; Qualtrics, a buyer expertise administration firm, was taken non-public by Silver Lake and CPP Investments for $12.5B; Coupa, a spend administration platform, was taken non-public by Thoma Bravo for $8B; New Relic, a monitoring and observability platform, was acquired by Francisco Companions and TPG for $6.5B; Alteryx, a knowledge analytics platform, was taken non-public by Clearlake Capital and Perception Companions for $4.4B; Salesloft, a income orchestration platform, was acquired by Vista Fairness for $2.3B, which then additionally acquired Drift, an AI chatbot developer for buyer expertise; Databricks, a supplier of knowledge lakehouses, acquired MosaicML, an AI improvement platform, for $1.3B (and a number of other different corporations, for decrease quantities like Arcion and Okera); Thoughtspot, a knowledge analytics platform, acquired Mode Analytics, a enterprise intelligence startup, for $200M; Snowflake, a supplier of knowledge warehouses, acquired Neeva, a shopper AI search engine, for $150M; DigitalOcean, a cloud internet hosting supplier, acquired Paperspace, a cloud computing and AI improvement startup, for $111M; NVIDIA, a chip producer for cloud computing, acquired OmniML, an AI/ML optimization platform for the sting.
IPOs?
In public markets, AI has been a sizzling pattern. The “Magnificent Seven” shares (Nvidia, Meta, Amazon, Microsoft, Alphabet, Apple and Tesla) gained a minimum of 49% in 2023 and powered the general inventory market increased.
Total, there may be nonetheless a extreme dearth of pure-play AI shares in public markets. The few which can be accessible are richly rewarded – Palantir inventory jumped 167% in 2023.
This could bode nicely for a complete group of AI-related pre-IPO startups. There are plenty of corporations at important quantities of scale within the MAD area – at the start Databricks, but additionally quite a few others together with Celonis, Scale AI, Dataiku* or Fivetran.
Then there’s the intriguing query of how OpenAI and Anthropic will take into consideration public markets.
Within the meantime, 2023 was a really poor 12 months by way of IPOs. Solely a handful of MAD-related corporations went public: Klaviyo, a advertising automation platform, went public at a $9.2B valuation in September 2023 (see our Klaviyo S-1teardown); Reddit, a forum-style social networking platform (which licenses its content material to AI gamers) , went public at a $6.4B valuation in March 2024; Astera Labs, a semiconductor firm offering clever connectivity for AI and cloud infrastructure, went public at a $5.5B valuation in March 2024.