If a fintech agency with textual content information at their disposal isn’t utilizing it to make use of pure language processing fashions – a department of synthetic intelligence that teaches machines to know, analyze, and generate human language – they’re lacking out.
Pure language processing fashions or NLP can and must be employed often to evaluate a agency’s inner and exterior textual content materials to know the emotions of the shoppers in addition to these of workers. It may also be used to establish vital themes or enterprise tendencies for the corporate to evaluate and combine into their enterprise technique.
That is significantly so with the emergence of generative AI, making pure language processing capabilities extra highly effective than ever.
That’s the clear message from information scientist Sumedha Rai in an interview with Fintech Nexus in addition to in shows at two latest conferences in New York Metropolis this spring – the AI in Finance Summit and MLConf 2024 gathering of AI and machine studying specialists.
Nevertheless, these are simply two of the outcomes that companies can get out of ongoing textual content evaluation through NLP fashions.
Rai provides that such NLP instruments, used along with different machine studying and AI options, may also be used to quickly summarize and translate paperwork, perceive vital tags in textual content information, personalize interactions with prospects, and catch fraudsters by choosing up anomalies of their communications.
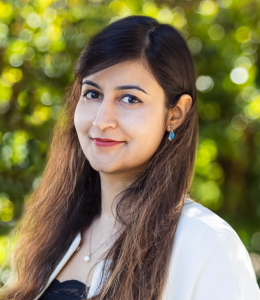
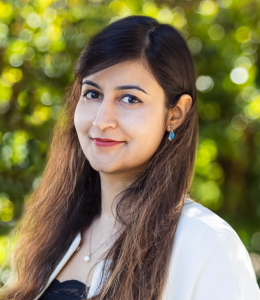
Rai is a senior information scientist at a micro-investment agency in New York Metropolis, the place she spends quite a lot of time analyzing person sentiment and themes, reviewing information to help in funding selections, and creating fraud prevention fashions. She additionally researches with the Heart for Knowledge Science and different affiliated departments at New York College.
She notes that maybe a very powerful profit that comes with common textual content evaluation through NLP – apart from higher effectivity — is that “folks (workers) may have much more time to consider the artistic stuff,” associated to product growth and one’s enterprise technique, which is a definite aggressive benefit.
Textual content related for NLP evaluation or summarization consists of every part from buyer suggestions, postings, complaints, social media feedback, emails and survey outcomes to transaction information, firm web site and inner information, worker communications, claims calls, agent suggestions, regulatory, compliance, and authorized information.
The advantages of quarterly or ongoing evaluation of such texts through NLP, Rai says, is that fintech companies can extra simply customise providers, construct higher chatbots, detect fraud, summarize and translate world compliance and regulatory paperwork, and achieve a greater understanding of worker satisfaction ranges.
One kind of textual content evaluation – utilizing NLP for subject modeling – can be utilized to trace the matters which might be uppermost within the minds of 1’s prospects – together with what they like or don’t like a couple of product — and is an exercise that Rai believes could also be underutilized by many fintech companies.
Utilizing this system, “Fintech companies ought to take into account all of their issues and challenges and see how a lot sign they’ve acquired for these issues within the type of textual content. They need to then leverage NLP evaluation of textual content information to assist remedy many of those points,” Rai says.
NLP fashions that may help with this train embody Latent Semantic Evaluation (LSA), Latent Dirichlet Allocation (LDA), LDA2vec, and BERTopic and its completely different variations although, for fintech companies specifically, utilizing FinBERT, a transformer mannequin that was particularly pretrained on monetary textual content, can also be an ideal selection.
Amongst these mannequin decisions, nevertheless, Rai is especially keen on the BERT fashions as a result of they’re bi-directional in design and seize context primarily based on this bi-directionality.
“They (BERT fashions) even have contextual embeddings, which allow the fashions to know a phrase by contemplating all different phrases round it and consider the context for every prevalence of a given phrase,” Rai says.
She provides: “Moreover, we now have entry to highly effective phrase embeddings from GenAI fashions, a few of that are freely downloadable. Nevertheless, BERT is a good selection for establishing a baseline when working with LLMs, significantly when working with monetary textual content.”
Rai additionally highlighted the significance of constructing full use of Named Entity Recognition (NER), a subfield of NLP that pertains to tagging textual content in order that named entities – particular person phrases, phrases, or sequences of phrases – will be simply categorized.
“NER is a base know-how that could be very underused however, in actual fact, will be employed in a number of methods to higher perceive what entities prospects are most occupied with, permitting you to higher tailor your communications with them,” Rai says.
She notes that NER evaluation offers us a technique to extract all important info loads sooner from a big physique of textual content and it may be used to flag dangerous interactions or anomalies that will point out potential fraud. On this means, it performs a pivotal position in a single’s ongoing sentiment evaluation and textual content classification.
One significantly useful characteristic, says Rai, is NER’s potential to assist one “eyeball compliance paperwork actually quick,” in order that one can shortly extract key info from prolonged paperwork and evaluate it later in an environment friendly method.
With the introduction of Generative AI fashions, Rai says, fintech companies now have entry to a robust instrument for textual content evaluation the place minimal coding is concerned, when utilizing the out-of-box answer straight. Nevertheless, the tradeoff could also be within the stage of accuracy that could be misplaced in utilizing out-of-the-box Gen AI fashions versus superb tuning a mannequin for particular duties.
“Generative AI fashions are pre-trained and so, for a easy textual content evaluation, a pre-trained mannequin can usually do the job,” Rai says, including that with a number of generative AI fashions to select from, she favors the convenience of use of Chat GPT which continues to enhance in accuracy and likewise has simply accessible APIs to combine the GPT fashions into code.
She additionally finds Meta’s LLAMA fashions – LLAMA 3 specifically – to be highly effective and useful and it’s free to make use of.
Nevertheless, Rai warns that fintech companies do must understand that there are dangers in utilizing out-of-the-box generative AI fashions.
“No delicate or buyer information must be fed to those fashions. These are hosted programs and the info goes out of your native machines and to a server the place the mannequin resides,” Rai says noting that the info from interactions will be analyzed by the businesses making the LLMs to enhance efficiency and reliability of their programs.
“Even if you’re utilizing the enterprise model of those fashions, I’d nonetheless be sure that your information has been stripped of all personally identifiable info (PII) earlier than it’s fed right into a mannequin or used to question the mannequin,” Rai says.
Evaluating fashions for bias, discrimination, information safety, information privateness, hallucinations, and respectful content material creation can also be key, Rai says, and begins with taking a look at what kind of information you’re ingesting into the mannequin, ensuring all courses, genders, and geographies are represented and likewise by using a various crew of individuals to work on fashions versus just one individual.
More and more, Rai says, some fintech companies are hiring purple groups from the skin of their firm to conduct an intensive evaluation and to make sure that a agency’s working fashions have been “de-biased.” will not be producing biased outcomes that may end up in discriminatory practices.
One Gen AI time saver that Rai significantly appreciated concerned asking Chat GPT to create a brand, tagline, and launch press launch for a fantasy fintech agency.
“The outcomes had been spectacular,” Rai mentioned, noting that on an ongoing foundation, Chat GPT continues to enhance and to impress.